New Study on Sex Discrimination Reveals Very Good News
We seem to have made a lot of progress when it comes to sex discrimination.
by Lee Jussim, Connors Institute & Rutgers University
We have some really good news regarding sex discrimination in the labor market from a recent meta-analysis that examined 85 audit studies (the U.S. being the country these studies most frequently took place in) assessing workplace sex discrimination. This analysis included over 360,000 job applications conducted from 1976 to 2020.
A meta-analysis is an array of methods used to combine and summarize results from many studies, in order to figure out what the big picture is. It is sorta like taking an average (though in practice a bit more complicated). Has prior research consistently found the effect? If so, how big or small is it? Is the effect different for different types of studies on the same topic? How much have researcher or publication biases distorted the main findings?
Audit studies are one of the strongest methodological tools available for assessing discrimination. First, they are experiments, so they are excellently designed to test whether bias causes unequal outcomes. This is in sharp contrast to studies that merely identify “gaps” (inequality in some outcome across groups). These “gap” studies are routinely interpreted as evidence of discrimination, even though discrimination is only one of many possible explanations for gaps. In audit studies, targets who are otherwise identical (e.g., identical or equivalent resumes) differ on some demographic characteristic and apply for something (such as a job). Thus if Bob receives more callbacks or interviews than Barbara, the result can be attributed to sex discrimination. Second, they are conducted in the real world, for example, by having fictitious targets apply for advertised jobs. These two strengths—strong methods for causal inference and tests conducted in the real world—render audit studies one of the best ways to test for discrimination.
The audit studies in the meta-analysis examined whether otherwise equivalent men or women were more likely to receive a callback after applying for a job.
The meta-analysis had two unique strengths that render it one of the strongest meta-analyses on this, or any other, topic yet performed. First, the methods and analyses were pre-registered, thereby precluding the undisclosed flexibility that can permit researchers to cherry pick findings to support a narrative and enhance chances of publication. Few existing meta-analyses in psychology on any topic have been pre-registered. Second, they hired a “red team”1—a panel of experts paid to critically evaluate the research plan. In this particular case, the red team included four women and one man, three had expertise in gender studies, one was a qualitative researcher, and one librarian (for critical feedback regarding the comprehensiveness of the literature search). The red team critically evaluated the proposed methods before the study began, and the analyses and draft of the report after the study was conducted.
Pre-registration refers to preparing a written document stating how some study will be conducted and analyzed, including how hypotheses will be tested, before the research is actually conducted. This was one of the reforms that emerged from psychology’s Replication Crisis. It prevents researchers from conducting some study, performing ten zillion analyses and cherry picking a few about which they can tell a good story without saying what they did, thereby conveying the false impression that they are brilliant and that their theory, hypotheses, and results are credible. It also prevents researchers from failing to report studies that found no hypothesized effects or relationships at all. In short, it dramatically reduces researchers’ ability to make themselves look like sharpshooters when they are just making stuff up like this:
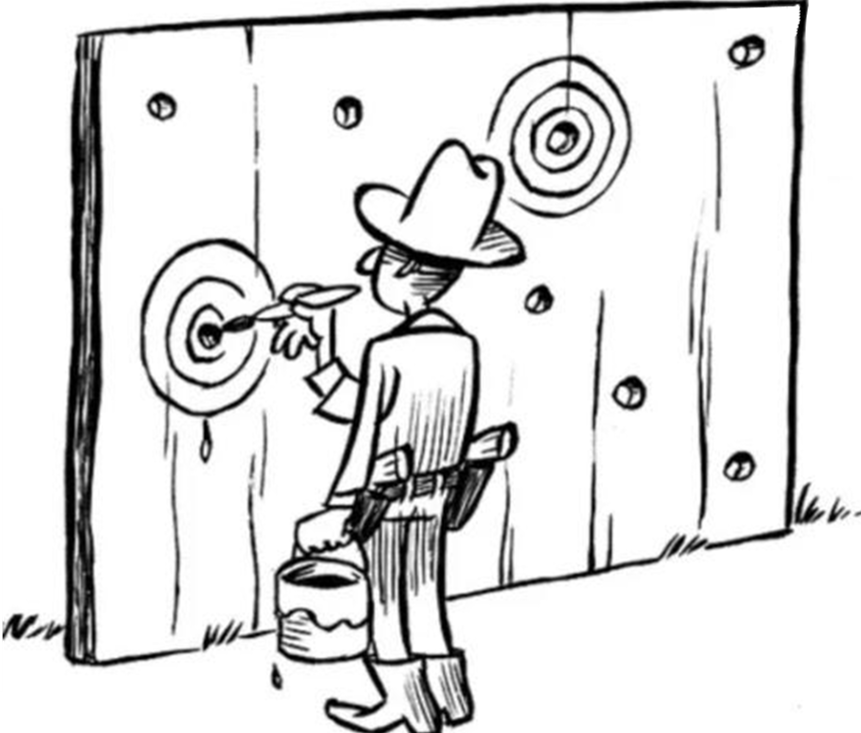
Some Key Results
Overall, men were statistically significantly less likely than women to receive a callback. Men were, overall, 9% less likely to receive a callback than were women.
Men were much less likely than women to receive a callback for female-typed jobs. Men were 25% less likely than women to receive a callback for these jobs.
There were no statistically significant2 differences in the likelihood of men or women receiving callbacks for male-typical or gender-balanced jobs. English translation: callbacks were nondiscriminatory.
Analysis of the discrimination trend over time found that women were somewhat disadvantaged in studies conducted before 2009 after which the trend reversed, such that there was a slight tendency to favor women after 2009.
The research team also had both laypeople and academics predict the results of their meta-analysis. All vastly overestimated the amount of bias favoring men and erroneously predicted that that bias persisted into the present, although laypeople were somewhat more inaccurate than were academics. The overestimates were dramatic, with laypeople estimating that men were three times more likely to be called back than women even in the most recent period they studied (2009-2020). Academics were not quite as bad, but still pretty awful given that their credentials should confer some higher level of knowledge about these sorts of things. Academics estimated that men were twice as likely as women to be called back for jobs in the 2009-2020 period (for both groups, the overestimates were more extreme the further back they went).
Academic expertise made no difference—academics who had published on gender were just as inaccurate as those who had not.
The Failure of the Academic Experts
In some sense, these academics were asked to make a “prediction”—one regarding how the results of the not-yet-performed meta-analysis would turn out. But in another sense, it was no prediction at all—it was a test of how well they knew the findings in a research area in which they were supposedly experts. That is because the meta-analysis was not really a “prediction” about “future” findings. It was a summary of what has been found by past research going back to 1976. You might think that experts would know the findings in exactly the area in which they supposedly have expertise. These academics dramatically failed that test.
Keep in mind how extreme the failure was (predicting men were twice as likely to get callbacks when in fact men were 9% less likely to receive callbacks). Now think about all of these academic experts promoting inaccurate information to other academics, the wider public, and their students. Not good at all.
This Analysis Has Limitations
The meta-analysis did not address every conceivable type of sex discrimination. It is possible that there is much more evidence of sex discrimination in other contexts (e.g., actual hiring, promotions, salaries, or different types of jobs).
It is very difficult for experimental studies such as those in this meta-analysis to examine actual hiring decisions because one would need real people (rather than, e.g., resumes) who answer callbacks, go for interviews, and ultimately do or do not receive jobs. The vastly greater time and attention it would require of businesses doing the hiring of fake applicants also would render such studies probably undoable because of ethical issues (they would be exploiting large investments of company time and effort for the scientists’ research purposes).
Thus, experimental audit studies are probably about as good as we can do to assess levels of sex (or other) discrimination in the real world.
Conclusion
As the authors put it in their conclusion, their main findings are very good news indeed for women:
“Contrary to the beliefs of laypeople and academics revealed in our forecasting survey, after years of widespread gender bias in so many aspects of professional life, at least some societies have clearly moved closer to equal treatment when it comes to applying for many jobs.”
Their results, however, do raise an interesting question: Why do so many people, especially academics who should know better, so wildly overestimate sex discrimination? That, however, is a question for an essay another day.
Lee Jussim is a Connors Institute advisory council member and psychologist at Rutgers University.
Essay originally published by Unsafe Science
As per Wikipedia: A red team is a group that pretends to be an enemy, attempts a physical or digital intrusion against an organization at the direction of that organization, then reports back so that the organization can improve their defenses.
Don’t confuse “statistical significance,” a technical academic term, with “importance.” Statistical significance has a highly technical meaning that I am not going to bother with here involving null hypotheses and probabilities. Without spending a few semesters in good statistics courses, suffice it to say statistical significance functions as a threshold whereby researchers are greenlighted to take seriously as “real” whatever difference or relationship the term is applied to. This is to be contrasted with typically minor differences that result from noise or randomness, which does not give researchers the green light to take their results seriously.